Genomic selection and reproductive efficiency in dairy cattle
Thomas E. Spencer1, Peter J. Hansen2, John B. Cole3, Joseph Dalton4, and Holly Neibergs1
1Department of Animal Sciences, Washington State University, Pullman, WA 99164; 2Department of Animal Sciences, University of Florida, Gainesville, FL 32611; 3USDA‐ARS, Animal Genomics and Improvement Laboratory, Beltsville, MD 20705; 4Department of Animal and Veterinary Science, University of Idaho, Caldwell, ID 83605
Introduction
Average milk yield per lactation nearly doubled in U.S. Holstein cows between 1950 and 2000 (Figure 1). More than half of this progress resulted from genetic selection for milk yield (Dekkers and Hospital, 2002), because milk yield and composition have moderate heritability (20 to 40%) (Hayes et al., 2010; Kemper and Goddard, 2012). During the same period, cow fertility declined in US dairy cattle (reviewed by Washburn et al., 2002; Diskin et al., 2006; Diskin and Morris, 2008b; Hansen, 2011). Reproductive status of US Holstein and Jersey cattle between 1996 and 2006 was evaluated in 2009 (Norman et al., 2009). During that 10‐year period, conception rate (CR), defined as the probability of a successful outcome of individual breeding services (Averill et al., 2004), declined by 3% and 4% for all services in Holsteins and Jerseys, respectively. By 2006, overall CR was 30% in Holsteins and 35% in Jerseys. The number of breedings per lactation increased in both Holsteins and Jerseys, and the days from calving to last breeding increased 10 or 11 days in Holsteins and Jerseys, respectively. Conception rate decreased in both Holstein and Jersey cattle as more breedings took place during lactation or as the number of calvings increased. Primiparous Holsteins had a CR of 34% after the first breeding, which declined to 26% by the fifth breeding. In contrast, mean CR for US Holstein and Jersey replacement heifers was 56.3% and 52.2%, respectively (Kuhn et al., 2006). In 2010, reproductive management data from 85 Holstein dairies in 4 regions of the US were analyzed and found that 21‐day pregnancy rates (PR) to artificial insemination (AI) were similar among regions and averaged only 18.5% (Moeller et al., 2010). Thus, dairy cattle continue to have problems with fertility or reproductive efficiency that affects the sustainability and profitability of US dairy production enterprises.
Given the decline in important reproductive traits, more selection emphasis has been placed on fertility in recent years. For example, days open (DO), which is used to calculate PR for genetic evaluations, increased by 37 days for Holsteins from 1960 through 2000; approximately 75% of that increase in DO was attributed to genetics as a consequence of selection for greater milk yield traits. A negative genetic correlation between milk yield and fertility exists in dairy cattle (VanRaden et al., 2004; Pritchard et al., 2013). Although fertility traits have low heritability (1 to 10%;( Sun et al., 2010), the large improvement in milk yield was accompanied by a decline in fertility (Washburn et al., 2002; Hare et al., 2006; Norman et al., 2009). The genetic correlation between milk yield and interval between calving and first insemination is 0.43 (Sun et al., 2010). The genetic correlation of cow fertility, as measured by DO, with milk yield is about 0.35 (VanRaden et al., 2004). Thus, selection on milk yield traits without concomitant selection for fertility is proposed to be the major cause of the decline in cow fertility between 1960 and 2000 in spite of relatively low heritabilities for reproductive traits (Veerkamp and Beerda, 2007). For example, the heritability for daughter pregnancy rate (DPR), the dairy fertility trait most widely measured in the United States, has been estimated at 4% (VanRaden et al., 2004). Fortunately, historical trends in fertility have started to recover because of the incorporation of genetic merit for productive life (PL) in 1994 and DPR in 2003 as well as the change in breeding strategies from natural service to ovulation synchronization programs coupled with AI that decrease DO. Poor fertility of dairy cattle involves a myriad of different aspects including anovulation, inadequate expression of estrus, irregular estrous cycles, and pregnancy loss after insemination (Lucy, 2007). The majority of pregnancy loss occurs during the first month and embryonic period of gestation (Hansen, 2011). The main factors implicated in pregnancy success (or loss) in dairy cows are those of genetic, physiological, endocrine, environmental, and disease origin. The reader is referred to several recent reviews on the contributions of oocyte quality, progesterone insufficiency, sperm damage, oviductal and uterine environment, disease, negative energy balance, and maternal metabolism to fertility problems in lactating dairy cattle (Lucy, 2007; Diskin and Morris, 2008a; Hansen, 2011;, LeBlanc, 2014; Wiltbank et al., 2014). Although a myriad of gene expression profiling studies have been conducted in dairy and beef cattle, we still do not really understand the gene networks and biological pathways that regulate the physiological processes that control maternal fertility (i.e., estrous behavior, hypothalamic‐pituitary function, ovarian function [oogenesis and ovulation]), oviductal and uterine function, conceptus development, and maternal metabolism (Bauersachs and Wolf, 2012l Lonergan and Forde, 2014). New management tools are needed in cattle to decrease embryonic mortality and pregnancy loss, thereby increasing overall herd fertility, reproductive and productive longevity, and sustainability of dairy enterprises. Sufficient genetic variability exists within major breeds for fertility traits that are complex and polygenic with low heritability. Selection for those fertility traits, however, relies on genomic selection strategies that require many different markers developed from analysis of carefully phenotyped animal populations. Fortunately, advent of high throughput “next generation” DNA sequencing (NGS) and other genomic technologies, such as single nucleotide polymorphism (SNP) genotyping, is useful to identify genes to improve reproductive efficiency in dairy cattle without negatively affecting desirable production traits, such as milk yield. Genetic selection for reproduction and health traits as well as new reproductive and genomic technologies is expected to continue improving dairy cow fertility and productivity (Lucy, 2007; Schefers and Weigel, 2012). The objective of this review is to highlight how genetics and genomic selection may be used to increase reproductive efficiency and fertility in dairy cattle.
Genetics of fertility
Improvements in fertility of both heifers and lactating cows would be of great benefit to the U.S. dairy industry. Despite the low heritability for common fertility traits, substantial genetic effects have been reported and significant genetic variation for fertility exists in lactating cows (Veerkamp and Beerda, 2007). A genome‐wide analysis (GWAS) of 31 production, health, reproduction and body conformation traits in contemporary Holstein cows was conducted recently, and the results supported the hypothesis that most traits are polygenic, each gene having a small effect on the phenotype (Cole et al., 2011). Genetic causes influencing fertility include chromosomal defects, individual genes and genetic interactions (VanRaden and Miller, 2006). The advent of new genomic technologies and improvements in NGS allows for the discovery of new genetic markers useful to improve fertility in dairy cattle using genomic selection strategies.
Sequencing and analysis of the approximately 2.87 gigabase pairs Bos taurus genome indicates that it contains more than 22,000 genes. Availability of the cattle genome sequence has allowed development of commercially available genome‐wide SNP assays that can be used to identify genomic regions and pathways associated with complex polygenic traits such as disease resistance and susceptibility (Matukumalli et al., 2009; Settles et al., 2009). The SNPs are estimated to account for 84% of the variation in gene expression in animals. If present in the coding region of genes, SNPs can affect protein production or function. The SNPs not in the coding region may still affect protein production by modulating gene splicing, transcription factor binding, and mRNA degradation of noncoding RNAs. For complex traits, individual SNPs usually work in coordination with other SNPs. The next generation of cattle SNP assays currently available consists of 2 different high density cattle SNP panels available from Illumina (777K) and Affymetrix (640K) for identifying and precise mapping of loci associated with desirable traits.
It is now recognized in humans that structural genetic variation involves more DNA sequences than the sequences represented by SNP (Conrad et al., 2010). The cattle sequence and recent NGS studies revealed more complex mutations such as segmental duplications that make up approximately 3% of the cattle genome with the majority (76%) of those corresponding to complete or partial gene duplications (Bickhart et al., 2012). The duplicated genes typically encode proteins with functions that involve interactions with the external environment; however, the majority of segmental duplications within the cattle genome are structural DNA variants known as copy number variants (CNV). The CNVs are defined as DNA fragments 1,000 nucleotides or longer that vary in number when the tested genome is compared with a reference genome (Feuk et al., 2006) and may involve DNA duplications, deletions, inversions, and translocations. They are estimated to account for 18% of the variation in gene expression in animals. The CNVs may be inherited or occur de novo during gametogenesis, even in a relatively inbred population (Egan et al., 2007). In cattle, over 850 CNV loci have been identified in 538 cattle genes that cover 22 megabase pairs (Mbp) of the genome (Bae et al., 2010; Fadista et al., 2010). Twenty percent of the CNV identified in cattle are associated with segmental duplications and 30% with genes. It is not known if fertility traits are associated with CNVs, but an estimated 84% and 18% of the variation in gene expression in humans is associated with SNPs and CNV, respectively (Stranger et al., 2007). Thus, interrogation of both forms of variation is important for a comprehensive evaluation of the genomic regions associated with phenotypes.
Availability of genome‐wide SNP assays make association analysis the most powerful approach for identifying loci associated with complex traits (Risch and Merikangas, 1996). This method of analysis relies upon linkage disequilibrium or the non‐random association of alleles at 2 or more loci to detect associations between neutral markers and casual loci. Association studies do not require structured breed crosses or pedigreed populations of cattle, but instead use a sampling of the independent meiosis present in a cattle population. The studies also utilize high‐throughput highdensity SNP genotyping assays that dramatically reduce costs and increase study power. Use of a high density SNP assay is very efficient to identify loci of large effects that are associated with production traits in cattle (Settles et al., 2009; Zanella et al., 2011).
Daughter pregnancy rate (DPR)
Genetic improvement of female fertility can be achieved by indirect selection for productive life or body condition score and direct selection for DPR, the only measure of female fertility used for genetic evaluation of dairy sires. In the U.S., introduction of genetic evaluations for PL in the mid‐1990s provided the first opportunity to improve female fertility through genetic selection (VanRaden and Klaaskate, 1993). Although indirect selection tools can be helpful, direct selection for improved fertility is more desirable. Thus, genetic evaluations for DPR were introduced in 2003 (VanRaden et al., 2004). The DPR involves use of DO, which is computed from reported breeding dates for current cows and from calving interval for historical cows, and these are subsequently transformed into 21‐day PR that is a common, timely measure of reproductive efficiency on dairy farms. The best and worst available Holstein sires differ by 7.2% in DPR. Because a 1% difference in PR corresponds to approximately 4 DO (VanRaden et al., 2004), daughters of the best and worst DPR sires differ by roughly 29 DO per lactation. Mean DPR between sires in the top and bottom deciles is 4.9%, which corresponds to a difference of 20 DO per lactation. During the past 10 years, DPR has been incorporated into all major selection indices used by U.S. dairy farmers with a relative weight of 6.9 to 12% of the total economic value. The lifetime net merit index (http://aipl.arsusda.gov/reference/nmcalc.htm) will be updated in December 2014 with the proposed weight on DPR decreasing from 11% to 7%. However, heifer and cow CR will be added to the index with weights of 2% each, which will maintain 11% of the overall emphasis on female fertility. The DPR is strongly correlated with a number of fertility traits including days to first service, CR and PR. Thus, producers can expect daughters of better DPR bulls to have improved fertility across a variety of management systems. Because of relatively low reliabilities, some bulls with better DPR will have over‐estimated DPR. Selection for DPR has resulted in genetic improvement (or at least stabilization) of female fertility (Figure 2) and could be used to increase PR by as much as 7% within a herd. Additional knowledge of genes and their products, however, is expected to make a substantial contribution to understanding and eventually improving fertility and other desirable traits in dairy cattle (Dawson, 2006).
Genetic estimates of fertility can be improved by genome‐wide SNP arrays. Utilization of the Bovine SNP50 chip from Illumina (San Diego, CA) improved reliability for DPR (Cole et al., 2011; Wiggans et al., 2011), but the poor heritability and polygenic nature of the trait has meant that improvements in reliabilities achieved by incorporation of genomic information was less than for other traits. Thus, although incorporation of information from the SNP50 chip increased reliability of DPR by 17% in Holsteins, this improvement was one of the least of the 12 traits examined (Wiggans et al., 2011). One possible way to improve accuracy of genomic estimates of fertility is to incorporate SNPs for specific genes involved in reproduction into genomic selection panels. The bovine genome contains over 20,000 genes, and over 14,000 of those do not contain a single SNP on the Bovine SNP50 chip (Michelizzi et al., 2011). Incorporation of candidate gene SNPs into genomic tests for reproduction would allow selection of causative SNPs or SNPs physically more close to causative SNPs. Such an approach has been successful for improving ability to detect genomic associations with disease (Amos et al., 2011). Many genes have been associated with reproduction in the dairy cow. Among these are SNPs related to in vitro fertilization or development, such as DNAJC27, FGF2, GHR, PGR, SPP1, STAT3, and STAT5A (Khatib et al., 2008a; Khatib et al., 2008b; Driver et al., 2009; Khatib et al., 2009b; Wang et al., 2009; Zhang et al., 2011), DPR (CAST; Garcia et al., 2006), sire CR including FGF2, ITGB5 and STAT5A (Feugang et al., 2009; Khatib et al., 2010), and calving interval (GHR; Waters et al., 2011), superovulation response (FSHR; Yang et al., 2010), twinning rate (IGF1; Kim et al., 2009), and incidence of still birth (NLRP9 and LEP; Ponsuksili et al., 2006; Brickell et al., 2010). The previously mentioned SNPs only represent a small portion of the genes involved in reproductive processes. Recent studies have revealed genes whose expression in tissues or cells of importance to reproduction vary with reproductive status; these genes are candidates for containing SNPs that impact fertility. For example, genes were identified that were differentially regulated in the brain of cows displaying strong estrus compared with those displaying weak estrus (Kommadath et al., 2011), in the endometrium of heifers which produced viable embryos compared with those which produced non‐viable embryos (Beltman et al., 2010), and in biopsies from embryos that resulted in live calves compared with embryos that died following embryo transfer (El‐Sayed et al., 2006). Genetic variants in the genes differentially expressed in the aforementioned studies and others may be responsible for differences in fertility among animals.
In order to evaluate effectiveness of SNPs in candidate genes for explaining genetic variation in DPR, semen was genotyped from 550 Holstein bulls of high or low DPR for 434 candidate SNPs (Cochran et al., 2013a). Three types of SNPs were evaluated: (1) SNPs previously reported to be associated with reproductive traits or physically close to genetic markers for reproduction; (2) SNPs in genes well known to be involved in reproductive processes; and (3) SNPs in genes differentially expressed between physiological conditions in a variety of tissues associated with reproductive function. A total of 40 SNPs were associated with DPR. Among these were genes involved in the endocrine system, cell signaling, immune function, and inhibition of apoptosis. A total of 10 genes were regulated by estradiol. In addition, 22 SNPs were associated with heifer CR, 33 with cow CR, 36 with productive life, 34 with net merit, 23 with milk yield, 19 with milk fat yield, 13 with milk fat percentage, 19 with milk protein yield, 22 with milk protein percentage, and 13 with somatic cell score. Allele substitution effect for SNPs associated with heifer CR, cow CR, PL, and net merit were in the same direction as for DPR. Allele substitution effects for several SNPs associated with production traits were correlated negatively with DPR. Nonetheless, 29 SNPs associated with DPR were not negatively associated with production traits. These SNPs are now being incorporated into genomic tests of reproduction and other traits such as the GeneSeek® Genomic Profiler™ (Neogen Agrigenomics, Lincoln, NE). Genes associated with DPR also are likely important for understanding reproduction. Given the large number of SNPs associated with DPR that were not negatively associated with production traits, it should be possible to select for DPR without compromising production.
The results of that study (Cochran et al., 2013a) support the idea that a candidate gene approach could be a successful method of determining markers for DPR. It was anticipated that, because the SNPs used for genotyping were specifically chosen for their function in reproductive processes, a larger proportion of them would be associated with reproductive traits than for production traits. Such a result was obtained. Of the 98 genes that met analysis criteria, 42 genes were associated with DPR, but only 23 were associated with milk yield. Moreover, all of the significant SNP effects for DPR were between 5 and 25 times greater than the largest marker effect from the Bovine SNP50 chip (Cole et al., 2011), probably because of differences in SNP selection between the 2 methods. The majority of SNPs on the Bovine SNP50 chip are between genes (63%) with over 14,000 genes represented by a SNP in close proximity, but not within the gene itself on the Bovine SNP50 chip (Michelizzi et al., 2011). In the current study, almost all of the SNPs examined were located in the coding region of the gene, and the remainder close physically to the coding region. Moreover, SNPs were chosen to maximize the probability that a change might occur in the characteristic of the protein encoded for the gene. Thus, it is likely that many of the SNPs that have large effects on DPR do so because they are causative SNPs resulting in changes in protein function. The remainder may represent linkages to causative SNPs. The SNPs identified in this study may be closer to the causative SNPs than the SNPs on the Bovine SNP50 chip.
Fertilization and embryo development
Fertilization and development of the pre‐implantation embryo is under genetic control. Existence of genes controlling fertilization and embryonic development indicates that some historical decline in fertility in dairy cattle may be the result of increased frequency of alleles that inhibit conceptus development (Lucy, 2001, Hansen, 2011). There are SNPs in cattle for which the beneficial allele for milk production is the less desirable allele for reproduction (Pimentel et al., 2011; Cochran et al., 2013a). Therefore, selection for production traits may have reduced the frequency of alleles that benefit fertility. In cattle, several SNPs have been associated with these traits. For example, SNPs in DNAJC27, FGF2, GHR, PGR, SPP1, STAT3, and STAT5A have been associated with in vitro fertilization (Khatib et al., 2008b; Driver et al., 2009; Khatib et al., 2009a; Khatib et al., 2009b; Khatib et al., 2009c; Wang et al., 2009; Cochran et al., 2013b). Furthermore, SNPs in DNAJC15, FGF2, GHR, PGR, PRLR, SERPINA15, STAT3, STAT5A, and SPP1 have been associated with in vitro development of embryos to the blastocyst stage (Khatib et al., 2008a; Khatib et al., 2008b; Driver et al., 2009; Khatib et al., 2009b; Wang et al., 2009; Zhang et al., 2011). There is a report that alleles for FGF2 and STAT5A also are associated with bull fertility (Khatib et al., 2010), but another group failed to find an effect of allelic variation in FGF2 or STAT5A on female fertility in vivo (Oikonomou et al., 2011). In contrast, (Al Naib et al. (2011) found that bulls with increased CR in vivo also had increased cleavage rates in vitro. Allelic SNP variants related to in vitro development also may be related to DPR and fertility in vivo. Recently, Cochran et al. (2013b) determined if any of the 434 SNPs previously studied (Cochran et al., 2013a) were associated with genetic variation in fertilization and early embryonic development. They produced embryos from 93 bulls using in vitro procedures and related cleavage rate and development of cleaved embryos to the blastocyst stage to the genotype for each SNP. Bulls were selected to have either large or small estimates for predicted transmitted ability for DPR, an estimate of future daughters’ fertility. The repeatability was 0.84 for cleavage rate and 0.55 for blastocyst development. No significant correlation was detected between DPR and either cleavage rate or blastocyst development. A total of 100 SNPs had a minor allele frequency sufficiently large (> 5%) to allow association analysis. Nine genes with SNPs were associated with cleavage rate (AVP, DEPP, EPAS1, HSD17B6, NT5E, SERPINE2, SLC18A2, TBC1D24, and a noncharacterized gene) and 12 genes with SNPs associated with blastocyst development (C1QB, FAM5C, HSPA1A, IRF9, MON1B, PARM1, PCCB, PMM2, SLC18A2, TBC1D24, TTLL3, and WBP1). Results indicate that in vitro cleavage rate and blastocyst development are under genetic control and highlight the potential importance of some previously unknown genes in these processes. Selection of cattle based on the genotype at one or more of these 19 loci may prove useful in conjunction with other genetic markers for improving fertility. Indeed, failure of fertilization and embryo cleavage to form a blastocyst is a cause of pregnancy loss in high milk‐producing dairy cattle (Hansen, 2011).
Genome‐wide association studies (GWAS)
Candidate gene studies have often produced inconclusive results, due largely to systematic under‐powering and lack of replication (Pasche and Yi, 2010). With the widespread availability of low‐cost, high‐density SNP panels, candidate gene studies are being complemented by GWAS, which can provide valuable insights into the genetic architecture of complex processes. A study of high‐density (> 250K SNP) human panels (Wilkening et al., 2009) concluded that there is sufficient coverage of individual genes and pathways that candidate gene studies are not necessary. In contrast, most dairy cattle currently are genotyped using a 50K SNP panel, which may not adequately cover rare gene variants or markers with only moderate effects on phenotypes. The latter point is particularly important because associations that are not detected may be valuable for understanding the biological basis of complex phenotypes (Cantor et al., 2010). Measures of fertility, such as DPR, are the phenotypic expression of complex genotypes affected by many loci (Cole et al., 2009). Fertility measurements have low heritabilities, and few loci with large effects on those traits have been identified (Cole et al., 2009; 2011).
No GWAS employing the high‐density bovine SNP panels (> 500K SNP) have been reported with a focus on fertility in dairy cows. Using a low‐density SNP panel, Sugimoto et al. (2013) investigated genetic effects on CR in Holstein cows. The DNA was collected from 4,362 Holstein cows and used to evaluate the estimated breeding value (EBV) for CR (Averill et al., 2004). The EBV is a genetic component obtained by subtracting an environmental component from a phenotype. The mean EBV for CR was 45.3 ± 3.5%. Next, the samples were stratified and grouped by CR with 192 low samples (CR < 41.1%) and 192 high samples (CR > 51%) and then genotyped for 54,001 SNPs using the Illumina Bovine SNP50 v1 panel. This analysis found 6 loci associated with CR on chromosomes 3, 5, 13, 18, and 28. The associated regions surrounding 2 significant SNPs, ARS‐BFGL‐NGS‐72055 (chromosome 3) and BTB‐01171634 (chromosome 28), did not include any known genes. The other 4 loci harbored 2 gap junction‐related genes, plakophilin 2 (PKP2) and cortactin‐binding protein 2 N‐terminal like (CTTNBP2NL), and 2 neuroendocrine‐related genes, SET domain containing 6 (SETD6) and calcium channel, voltage‐dependent, beta 2 subunit (CACNB2). Knockdown of Pkp2 or overexpression of CTTNBP2NL inhibited embryo implantation in mice. Additional experiments found that SETD6 is involved in the transcriptional regulation of GnRH, whereas CACNB2 controlled the secretion of FSH in cattle. The total allele substitution effect of these genes on CR was 3.5%. Moreover, these genes had favorable effects on the duration of DO, which was reduced by 8.5 days as the total allele substitution effect. Days open is an important fertility trait in cattle, and its additive genetic variance is 90.5 days. Therefore, genetic variants related to gap junctions and neuroendocrinology influence CR as well as DO.
Deleterious recessive lethal alleles
With the recent advent of genomic tools for cattle, several recessive conditions affecting fertility have been identified and selected against, such as deficiency of uridine monophosphate synthase, complex vertebral malformation, and brachyspina. In the Holstein breed, 2 major recessive defects affecting embryo or fetal survival have been detected. Deficiency of uridine monophosphate synthase (DUMPS; Robinson et al., 1984), a homozygous recessive condition, causes fetal death between 40 and 50 of gestation (Shanks and Robinson, 1989). Testing of AI sires for DUMPS and selecting against it has reduced the frequency of heterozygous sires and of homozygous recessive embryos, and has now almost eliminated DUMPS as a cause of infertility (VanRaden and Miller, 2006). Complex vertebral malformation is a lethal recessive condition that causes late fetal death in cattle. This defect was disseminated by widespread use of the Holstein sire, Carlin‐M Ivanhoe Bell (Thomsen et al., 2006). Availability of genomewide, high‐density SNP panels, combined with the typical structure of livestock populations, markedly accelerates the identification of genes and mutations that cause inherited defects (Charlier et al., 2008). This approach, combined with NGS, identified a 3.3 Kb deletion in the FANCI gene causing the brachyspina syndrome, a rare recessive genetic defect in Holstein cattle (Charlier et al., 2012). Despite the very small incidence of brachyspina (< 1 per 100,000), carrier frequency was as high as 7.4% in the Holstein breed.
VanRaden et al. (2011) recently reported the discovery of 5 haplotypes with deleterious effects on fertility in 3 breeds of dairy cattle, including 1 recessive in Ayrshire cattle, 2 recessives in Brown Swiss cattle, 3 in Holsteins, and 1 in Jerseys (VanRaden et al., 2011; Cooper et al., 2014). Similar methodology also has been used to identify novel recessives in other cattle populations (Fritz et al., 2013). The Jersey haplotype (JH1) was localized to the region between 11 and 16 Mbp on Bos taurus chromosome 15, and was determined to have a significant negative effect on fertility. Despite the relatively high carrier frequency (23.4%) in the population, no homozygous animals have been identified in the population, leading to the conclusion that JH1 is associated with early embryonic loss. The 5‐Mb region identified using SNP genotypes was therefore an ideal candidate for resequencing to identify the causal variant associated with this phenotype. The DNA sequence variation associated with the lethal haplotype JH1 was identified using targeted resequencing of heterozygous animals and the putative biological mechanisms underlying the JH1 phenotype (Sonstegard et al., 2013). By combining SNP analysis of whole‐genome sequences aligned to the JH1 interval and subsequent SNP validation, a nonsense mutation in CWC15 was identified as the likely causative mutation underlying the fertility phenotype. No homozygous recessive individuals were found in 749 genotyped animals, whereas all known carriers and carrier haplotypes possessed 1 copy of the mutant allele. This newly identified lethal has been responsible for a substantial number of spontaneous abortions in Jersey dairy cattle throughout the past half‐century and originated with 1 highly influential Jersey bull. With the mutation identified, selection against the deleterious allele in breeding schemes will reduce incidence of this defect in the population. Whole‐genome resequencing using NGS proved to be a powerful strategy to identify rapidly a previously mapped deleterious mutation in a known carrier of a recessive lethal allele.
Although the lethal effects discovered to date manifest themselves during later gestation or cause stillbirths, it is tempting to speculate that chromosomal abnormalities and specific lethal genes may cause failure of embryo cleavage to form a blastocyst or conceptus elongation that results in early embryonic mortality denoted by large or slightly delayed 21‐day return to estrus rates.
Copy number variation (CNV)
In addition to recessive lethal alleles and SNPs, CNV may also be a source of genetic variation that affects pregnancy loss in dairy cattle. A recent study identified a 660‐kb deletion encompassing 4 genes as a causative variant for a major fertility quantitative trait locus (QTL) in Nordic Red Cattle (Kadri et al., 2014). Of note, the deletion causes embryonic lethality because of the loss of RNASEH2B, which elicits embryonic death in mice lacking both alleles or null mice. Despite causing problems with fertility resulting from embryonic lethality, carrier frequency for the variant is 13%, 23%, and 32% of Danish, Swedish and Finnish Red Cattle, respectively, because the deletion has a strong positive effect on milk yield in those dairy cattle. This study supports the idea that that embryonic lethal mutations account for a non‐negligible fraction of the decline in fertility of dairy cattle and that positive effects on milk yield may account for part of the negative genetic correlation. This work adds to the evidence that embryonic lethals are at least, in part, responsible for the increase in fertility problems and conception failure observed in highly selected cattle populations. In man, largely deleterious alleles are typically rare, and hence homozygosity for such variants is exceptional in absence of consanguinity. In domestic animals, however, and as a result of intense selection and reduction in effective population sizes, a yet unidentified number of embryonic lethals may be segregating at low to moderate frequencies in most dairy cattle populations.
Genomic selection
Genomic selection can be defined as the study of differences between individual animals in the bovine genome sequence (SNP or CNV) that can be used to predict economically important traits, such as milk production, milk composition, health, fertility, or longevity (Meuwissen et al., 2001). Genetic information for a given calf, heifer, cow, or bull is compared with that of a reference population of older animals of the same breed. This reference population is composed of animals with known phenotypes that have been genotyped previously, and their phenotypic and genomic information are stored in an extensive database at the USDA‐ARS Animal Genomics and Improvement Laboratory (AGIL, Beltsville, MD). Dairy cattle are particularly well‐suited for genomic selection, because individual animals with high EBV have sufficient value to offset the costs of genomic testing, and because large reference populations of bulls with high reliability predictions of genetic merit exist for the purpose of estimating SNP effects or calculating genomic predicted transmitting abilities (GPTA). More than 350,000 dairy bulls, cows, heifers, and calves have genomic data in the AGIL database, and genomic predictions are available for Holstein, Jersey, and Brown Swiss cattle. Current cost of genomic testing is approximately $45 per animal with a low‐density (9K or 12K) chip, whereas the cost of medium‐density (54K) or high‐density chip (648K or 777K), genotyping is 2‐ to 4‐fold greater (GeneSeek, Lincoln, NE).
In North America, as in most countries with well‐developed genomic evaluation systems for dairy cattle, genotype information has been incorporated into genetic evaluation systems in a nearly seamless manner (Wiggans et al., 2011). Approximately 45,000 SNPs are used in routine genomic evaluations, and for animals that have been genotyped with lowdensity chips the remaining SNPs are imputed with 90 to 99% accuracy based on the medium‐ and high‐density genotypes of reference animals of the same breed (Boichard et al., 2012; VanRaden et al., 2013). In this manner, inexpensive low‐density genotyping of cows, heifers, and calves on commercial dairy farms is possible, and after genotype imputation, their GPTA values are of sufficient accuracy for selection and culling decisions (Weigel et al., 2010; Dassonneville et al., 2011).
In Holsteins, the average gains in reliability for production traits are 29, 31, and 23% for milk, fat, and protein, respectively, whereas gains for fitness traits are 22, 27, and 22% for DPR, somatic cell score, and duration of productive life, respectively. For protein yield, which has a heritability of approximately 30%, the amount of information provided by a young calf’s pedigree is equivalent to about 7 milkrecorded offspring, whereas the amount of information provided by the calf’s genotype is equivalent to about 34 additional daughters. In contrast, for DPR, which has a heritability of about 4%, the amount of information provided by the calf’s genotype is equivalent to about 131 additional daughters. Historically, the weak link in dairy cattle improvement programs has been the “dams to produce daughters” selection pathway, because of poor accuracy and low selection intensity (Van Tassell and Van Vleck, 1991). Reliability of traditional pedigree‐based PTA values for cows on commercial farms has tended to be small, and elevated rates of culling resulting from illness, injury, or infertility have typically prevented culling of genetically inferior replacement heifers. Culling rates, however, on modern, well‐managed free‐stall operations tend to be low, and widespread usage of gender‐enhanced (sexed) semen has generated an excess of replacement heifers. Thus, dairy producers have an opportunity to improve the genetic potential of their herds by culling inferior females at a young age and, more importantly, they can significantly reduce feed costs associated with rearing heifers that are unlikely to perform at a profitable level once they reach lactating age.
The discovery process for genomic selection and implementation into breeding strategies requires a 2‐stage approach in which animals with known phenotypes and genotypes are used in a training analysis to statistically establish the relationships between individual SNPs and trait variation and then the inferred equations that predict breeding value are validated in independent populations. All dairy breeding populations in the U.S. rely on using the most superior sires in the population to produce the next generation of sons, leading to high pedigree relationships between training and target populations. Simulation studies have shown that genomic selection improves the accuracy of selecting juvenile animals compared with traditional breeding methods and compared with selection using information from a few genes or quantitative trait loci (Veerkamp and Beerda, 2007). Improving dairy cow fertility by means of genetic selection is becoming increasingly important, because improved management alone cannot solve fertility problems.
Future possibilities and conclusions
Use of genetic technologies and genomic selection in livestock management will undoubtedly increase dairy cow fertility without sacrificing milk yield, thereby improving the economic and sustainability aspects of production enterprises. It is clear that genetic variability exists within the Holstein and Jersey breeds for important traits relating to fertility (Berry et al., 2007). Marker‐assisted selection will increase the rate of genetic progress for reproductive traits by increasing accuracy of selection and permitting selection in both genders at a younger age (Rohrer, 2004). The current genomic selection strategies for fertility traits rely mostly on sires, but markers developed for dam fertility traits are sorely needed. Reproduction‐enhancing technologies such as AI, embryo transfer and ovum pick‐up will have an important role in fully exploiting the potential of marker‐assisted selection (Georges and Massey, 1991; Humblot et al., 2010). Selective breeding has long been practiced to enrich for desirable DNA variation that influences livestock traits. Advent of new genetic engineering technologies allows for genetic variants to be directly introgressed into livestock genomes using modified meganuclease systems (Pennisi, 2013; Tan et al., 2013; Hsu et al., 2014). Transient exposure of livestock cells to sequence‐targeted editors stimulates homology‐directed repair to levels that eliminate need for transgene‐dependent selection. Use of oligonucleotide template enables efficient single nucleotide changes to the genome and permits the transmission of both natural and novel DNA sequence variants into naïve livestock breeds. Indeed, gene editing offers a powerful method for accelerating the genetic improvement of livestock. In summary, incorporation of genetic selection for reproduction and health traits as well as new technologies is expected to improve dairy cow fertility, reproductive efficiency, productivity, and sustainability.
Acknowledgments
This project is supported by Agriculture and Food Research Initiative Competitive Grant no. 2013‐68004‐20365 from the USDA National Institute of Food and Agriculture.
Literature cited
- Al Naib, A., J. P. Hanrahan, P. Lonergan, and S. Fair. 2011. In vitro assessment of sperm from bulls of high and low field fertility. Theriogenology 76:161‐167.
- Amos, W., E. Driscoll, and J. I. Hoffman. 2011. Candidate genes versus genome‐wide associations: which are better for detecting genetic susceptibility to infectious disease? Proc. Biol. Sci.‐The Royal Soc. 278:1183‐1188.
- Averill, T. A., R. Rekaya, and K. Weigel. 2004. Genetic analysis of male and female fertility using longitudinal binary data. J.Dairy Sci. 87:3947‐3952.
- Bae, J. S., H. S. Cheong, L. H. Kim, S. NamGung, T. J. Park, J. Y. Chun, J. Y. Kim, C. F. Pasaje, J. S. Lee, and H. D. Shin. 2010. Identification of copy number variations and common deletion polymorphisms in cattle. BMC Genomics 11:232.
- Bauersachs, S. and E. Wolf. 2012. Transcriptome analyses of bovine, porcine and equine endometrium during the preimplantation phase. Anim. Reprod. Sci. 134:84‐94.
- Beltman, M. E., N. Forde, P. Furney, F. Carter, J. F. Roche, P. Lonergan, and M. A. Crowe. 2010. Characterisation of endometrial gene expression and metabolic parameters in beef heifers yielding viable or non‐viable embryos on Day 7 after insemination. Reprod. Fertil. Dev. 22:987999.
- Bickhart, D. M., Y. Hou, S. G. Schroeder, C. Alkan, M. F. Cardone, L. K. Matukumalli, J. Song, R. D. Schnabel, M. Ventura, J. F. Taylor, J. F. Garcia, C. P. Van Tassell, T. S. Sonstegard, E. E. Eichler, and G. E. Liu. 2012. Copy number variation of individual cattle genomes using next‐generation sequencing. Genome Res. 22:778‐790.
- Brickell, J. S., G. E. Pollott, A. M. Clempson, N. Otter, and D. C. Wathes. 2010. Polymorphisms in the bovine leptin gene associated with perinatal mortality in Holstein‐Friesian heifers. J. Dairy Sci. 93:340‐347.
- Cantor, R. M., K. Lange, and J. S. Sinsheimer. 2010. Prioritizing GWAS results: A review of statistical methods and recommendations for their application. Am. J. Human Genet. 86:6‐22.
- Charlier, C., J. S. Agerholm, W. Coppieters, P. Karlskov‐Mortensen, W. Li, G. de Jong, C. Fasquelle, L. Karim, S. Cirera, N. Cambisano, N. Ahariz, E. Mullaart, M. Georges, and M. Fredholm. 2012. A deletion in the bovine FANCI gene compromises fertility by causing fetal death and brachyspina. PLoS One 7:e43085.
- Charlier, C., W. Coppieters, F. Rollin, D. Desmecht, J. S. Agerholm, N. Cambisano, E. Carta, S. Dardano, M. Dive, C. Fasquelle, J. C. Frennet, R. Hanset, X. Hubin, C. Jorgensen, L. Karim, M. Kent, K. Harvey, B. R. Pearce, P. Simon, N. Tama, H. Nie, S. Vandeputte, S. Lien, M. Longeri, M. Fredholm, R. J. Harvey, and M. Georges. 2008. Highly effective SNP‐based association mapping and management of recessive defects in livestock. Nat. Genet. 40:449‐454.
- Cochran, S. D., J. B. Cole, D. J. Null, and P. J. Hansen. 2013a. Discovery of single nucleotide polymorphisms in candidate genes associated with fertility and production traits in Holstein cattle. BMC Genet. 14:49.
- Cochran, S. D., J. B. Cole, D. J. Null, and P. J. Hansen. 2013b. Single nucleotide polymorphisms in candidate genes associated with fertilizing ability of sperm and subsequent embryonic development in cattle. Biol. Reprod. 89:69. Cole, J. B., P. M. VanRaden, J. R. O’Connell, C. P. Van Tassell, T. S. Sonstegard, R. D. Schnabel, J. F. Taylor, and G. R. Wiggans. 2009. Distribution and location of genetic effects for dairy traits. J. Dairy Sci. 92:2931‐2946.
- Cole, J. B., G. R. Wiggans, L. Ma, T. S. Sonstegard, T. J. Lawlor, Jr., B. A. Crooker, C. P. Van Tassell, J. Yang, S. Wang, L. K. Matukumalli, and Y. Da. 2011. Genome‐wide association analysis of thirty one production, health, reproduction and body conformation traits in contemporary U.S. Holstein cows. BMC Genom. 12:408.
- Conrad, D. F., D. Pinto, R. Redon, L. Feuk, O. Gokcumen, Y. Zhang, J. Aerts, T. D. Andrews, C. Barnes, P. Campbell, T. Fitzgerald, M. Hu, C. H. Ihm, K. Kristiansson, D. G. Macarthur, J. R. Macdonald, I. Onyiah, A. W. Pang, S. Robson, K. Stirrups, A. Valsesia, K. Walter, J. Wei, C. Tyler‐Smith, N. P. Carter, C. Lee, S. W. Scherer, and M. E. Hurles. 2010. Origins and functional impact of copy number variation in the human genome. Nature 464:704‐712.
- Cooper, T. A., G. R. Wiggans, D. J. Null, J. L. Hutchison, and J. B. Cole. 2014. Genomic evaluation, breed identification, and discovery of a haplotype affecting fertility for Ayrshire dairy cattle. J. Dairy Sci. 97:3878‐3882.
- Dawson, K. A. 2006. Nutrigenomics: feeding the genes for improved fertility. Anim. Reprod. Sci. 96:312‐322.
- Dekkers, J. C. and F. Hospital. 2002. The use of molecular genetics in the improvement of agricultural populations. Nat. Rev. Genet. 3:22‐32.
- Diskin, M. G. and D. G. Morris. 2008a. Embryonic and early foetal losses in cattle and other ruminants. Reprod. Domest. Anim. 43(Suppl. 2):260‐267.
- Diskin, M. G. and D. G. Morris. 2008b. Embryonic and early foetal losses in cattle and other ruminants. Reprod. Domest. Anim. Zuchthygiene 43 (Suppl. 2):260267.
- Diskin, M. G., J. J. Murphy, and J. M. Sreenan. 2006. Embryo survival in dairy cows managed under pastoral conditions. Anim. Reprod. Sci. 96:297‐311.
- Driver, A. M., W. Huang, S. Gajic, R. L. Monson, G. J. Rosa, and H. Khatib. 2009. Short communication: Effects of the progesterone receptor variants on fertility traits in cattle. J. Dairy Sci. 92:4082‐4085.
- Egan, C. M., S. Sridhar, M. Wigler, and I. M. Hall. 2007. Recurrent DNA copy number variation in the laboratory mouse. Nature Genet. 39:1384‐1389.
- El‐Sayed, A., M. Hoelker, F. Rings, D. Salilew, D. Jennen, E. Tholen, M.‐A. Sirard, K. Schellander, and D. Tesfaye. 2006. Largescale transcriptional analysis of bovine embryo biopsies in relation to pregnancy success after transfer to recipients. Physiol. Genom. 28:84‐96.
- Fadista, J., B. Thomsen, L. E. Holm, and C. Bendixen. 2010. Copy number variation in the bovine genome. BMC Genom. 11:284.
- Feugang, J. M., A. Kaya, G. P. Page, L. Chen, T. Mehta, K. Hirani, L. Nazareth, E. Topper, R. Gibbs, and E. Memili. 2009. Two‐stage genome‐wide association study identifies integrin beta 5 as having potential role in bull fertility. BMC Genom. 10:176.
- Feuk, L., A. R. Carson, and S. W. Scherer. 2006. Structural variation in the human genome. Nature reviews. Genetics 7:85‐97.
- Fritz, S., A. Capitan, A. Djari, S. C. Rodriguez, A. Barbat, A. Baur, C. Grohs, B. Weiss, M. Boussaha, D. Esquerre, C. Klopp, D. Rocha, and D. Boichard. 2013. Detection of haplotypes associated with prenatal death in dairy cattle and identification of deleterious mutations in GART, SHBG and SLC37A2. PLoS One 8:e65550.
- Garcia, M. D., J. J. Michal, C. T. Gaskins, J. J. Reeves, T. L. Ott, Y. Liu, and Z. Jiang. 2006. Significant association of the calpastatin gene with fertility and longevity in dairy cattle. Anim. Genet. 37:304‐305.
- Hansen, P. J. 2011. Challenges to fertility in dairy cattle: from ovulation to the fetal stage of pregnancy. Rev. Bras, Reprod. Anim. 35:229‐238.
- Hare, E., H. D. Norman, and J. R. Wright. 2006. Trends in calving ages and calving intervals for dairy cattle breeds in the United States. J. Dairy Sci. 89:365‐370. Hayes, B. J., J. Pryce, A. J. Chamberlain, P. J. Bowman, and M. E. Goddard. 2010. Genetic architecture of complex traits and accuracy of genomic prediction: coat colour, milk‐fat percentage, and type in Holstein cattle as contrasting model traits. PLoS Genet. 6:e1001139.
- Hsu, P. D., E. S. Lander, and F. Zhang. 2014. Development and applications of CRISPRCas9 for genome engineering. Cell 157:1262‐1278.
- Kadri, N. K., G. Sahana, C. Charlier, T. Iso‐Touru, B. Guldbrandtsen, L. Karim, U. S. Nielsen, F. Panitz, G. P. Aamand, N. Schulman, M. Georges, J. Vilkki, M. S. Lund, and T. Druet. 2014. A 660‐Kb deletion with antagonistic effects on fertility and milk production segregates at high frequency in Nordic Red cattle: additional evidence for the common occurrence of balancing selection in livestock. PLoS Genet. 10:e1004049.
- Kemper, K. E. and M. E. Goddard. 2012. Understanding and predicting complex traits: knowledge from cattle. Hum. Mol. Genet. 21:R45‐51.
- Khatib, H., W. Huang, D. Mikheil, V. Schutzkus, and R. L. Monson. 2009a. Effects of signal transducer and activator of transcription (STAT) genes STAT1 and STAT3 genotypic combinations on fertilization and embryonic survival rates in Holstein cattle. J. Dairy Sci. 92:6186‐6191.
- Khatib, H., W. Huang, X. Wang, A. H. Tran, A. B. Bindrim, V. Schutzkus, R. L. Monson, and B. S. Yandell. 2009b. Single gene and gene interaction effects on fertilization and embryonic survival rates in cattle. J. Dairy Sci. 92:2238‐2247.
- Khatib, H., C. Maltecca, R. L. Monson, V. Schutzkus, and J. J. Rutledge. 2009c. Monoallelic maternal expression of STAT5A affects embryonic survival in cattle. BMC genetics 10:13.
- Khatib, H., C. Maltecca, R. L. Monson, V. Schutzkus, X. Wang, and J. J. Rutledge. 2008a. The fibroblast growth factor 2 gene is associated with embryonic mortality in cattle. J. Anim. Sci. 86:2063‐2067.
- Khatib, H., R. L. Monson, W. Huang, R. Khatib, V. Schutzkus, H. Khateeb, and J. J. Parrish. 2010. Short communication: Validation of in vitro fertility genes in a Holstein bull population. J. Dairy Sci. 93:2244‐2249.
- Khatib, H., R. L. Monson, V. Schutzkus, D. M. Kohl, G. J. Rosa, and J. J. Rutledge. 2008b. Mutations in the STAT5A gene are associated with embryonic survival and milk composition in cattle. J. Dairy Sci. 91:784‐793.
- Kim, E. S., X. Shi, O. Cobanoglu, K. Weigel, P. J. Berger, and B. W. Kirkpatrick. 2009. Refined mapping of twinning‐rate quantitative trait loci on bovine chromosome 5 and analysis of insulin‐like growth factor‐1 as a positional candidate gene. J. Anim. Sci. 87:835‐843.
- Kommadath, A., H. Woelders, B. Beerda, H. A. Mulder, A. A. de Wit, R. F. Veerkamp, M. F. te Pas, and M. A. Smits. 2011. Gene expression patterns in four brain areas associate with quantitative measure of estrous behavior in dairy cows. BMC Genom. 12:200.
- Kuhn, M. T., J. L. Hutchison, and G. R. Wiggans. 2006. Characterization of Holstein heifer fertility in the United States. J. Dairy Sci. 89:4907‐4920.
- LeBlanc, S. J. 2014. Reproductive tract inflammatory disease in postpartum dairy cows. Animal 8(Suppl. 1):54‐63.
- Lonergan, P. and N. Forde. 2014. Maternalembryo interaction leading up to the initiation of implantation of pregnancy in cattle. Animal 8(Suppl. 1):64‐69.
- Lucy, M. C. 2001. Reproductive loss in highproducing dairy cattle: where will it end? J. Dairy Sci. 84:1277‐1293.
- Lucy, M. C. 2007. Fertility in high‐producing dairy cows: reasons for decline and corrective strategies for sustainable improvement. Soc. Reprod. Fertil. Suppl. 64:237‐254.
- Matukumalli, L. K., C. T. Lawley, R. D. Schnabel, J. F. Taylor, M. F. Allan, M. P. Heaton, J. O’Connell, S. S. Moore, T. P. Smith, T. S. Sonstegard, and C. P. Van Tassell. 2009. Development and characterization of a high density SNP genotyping assay for cattle. PLoS One 4:e5350.
- Meuwissen, T. H., B. J. Hayes, and M. E. Goddard. 2001. Prediction of total genetic value using genome‐wide dense marker maps. Genetics 157:1819‐1829.
- Michelizzi, V. N., X. Wu, M. V. Dodson, J. J. Michal, J. Zambrano‐Varon, D. J. McLean, and Z. Jiang. 2011. A global view of 54,001 single nucleotide polymorphisms (SNPs) on the Illumina BovineSNP50 BeadChip and their transferability to water buffalo. Int. J. Biol. Sci. 7:18‐27.
- Moeller, L. M., N. A. Michael, J. C. Dalton, and G. C. Lamb. 2010. Evaluating reproductive outcomes in the United States Holstein dairies. J. Dairy Sci. 93(E Suppl. 1):408.
- Norman, H. D., J. R. Wright, S. M. Hubbard, R. H. Miller, and J. L. Hutchison. 2009. Reproductive status of Holstein and Jersey cows in the United States. J. Dairy Sci. 92:3517‐3528.
- Oikonomou, G., G. Michailidis, A. Kougioumtzis, M. Avdi, and G. Banos. 2011. Effect of polymorphisms at the STAT5A and FGF2 gene loci on reproduction, milk yield and lameness of Holstein cows. Res. Vet. Sci. 91:235‐239.
- Pasche, B. and N. Yi. 2010. Candidate gene association studies: successes and failures. Current Opin. Genet. Dev. 20:257‐261.
- Pennisi, E. 2013. The CRISPR craze. Science 341:833‐836.
- Pimentel, E. C., S. Bauersachs, M. Tietze, H. Simianer, J. Tetens, G. Thaller, F. Reinhardt, E. Wolf, and S. Konig. 2011. Exploration of relationships between production and fertility traits in dairy cattle via association studies of SNPs within candidate genes derived by expression profiling. Anim. Genet. 42:251‐262.
- Ponsuksili, S., R. M. Brunner, T. Goldammer, C. Kuhn, C. Walz, S. Chomdej, D. Tesfaye, K. Schellander, K. Wimmers, and M. Schwerin. 2006. Bovine NALP5, NALP8, and NALP9 genes: assignment to a QTL region and the expression in adult tissues, oocytes, and preimplantation embryos. Biol. Reprod. 74:577‐584.
- Pritchard, T., M. Coffey, R. Mrode, and E. Wall. 2013. Genetic parameters for production, health, fertility and longevity traits in dairy cows. Animal 7:34‐46.
- Risch, N. and K. Merikangas. 1996. The future of genetic studies of complex human diseases. Science 273:1516‐1517.
- Robinson, J. L., D. B. Dombrowski, G. W. Harpestad, and R. D. Shanks. 1984. Detection and prevalence of UMP synthase deficiency among dairy cattle. J. Hered. 75:277‐280.
- Schefers, J. M. and K. A. Weigel. 2012. Genomic selection in dairy cattle: Integration of DNA testing into breeding programs. Anim. Front. 2:4‐9.
- Settles, M., R. Zanella, S. D. McKay, R. D. Schnabel, J. F. Taylor, R. Whitlock, Y. Schukken, J. S. Van Kessel, J. M. Smith, and H. Neibergs. 2009. A whole genome association analysis identifies loci associated with Mycobacterium avium subsp. paratuberculosis infection status in US holstein cattle. Anim. Genet. 40:655662.
- Shanks, R. D. and J. L. Robinson. 1989. Embryonic mortality attributed to inherited deficiency of uridine monophosphate synthase. J. Dairy Sci. 72:3035‐3039.
- Sonstegard, T. S., J. B. Cole, P. M. VanRaden, C. P. Van Tassell, D. J. Null, S. G. Schroeder, D. Bickhart, and M. C. McClure. 2013. Identification of a nonsense mutation in CWC15 associated with decreased reproductive efficiency in Jersey cattle. PLoS One 8:e54872.
- Stranger, B. E., M. S. Forrest, M. Dunning, C. E. Ingle, C. Beazley, N. Thorne, R. Redon, C. P. Bird, A. de Grassi, C. Lee, C. Tyler‐Smith, N. Carter, S. W. Scherer, S. Tavare, P. Deloukas, M. E. Hurles, and E. T. Dermitzakis. 2007. Relative impact of nucleotide and copy number variation on gene expression phenotypes. Science 315:848‐853.
- Sugimoto, M., S. Sasaki, Y. Gotoh, Y. Nakamura, Y. Aoyagi, T. Kawahara, and Y. Sugimoto. 2013. Genetic variants related to gap junctions and hormone secretion influence conception rates in cows. Proceedings of the National Academy of Sciences of the United States of America 110(48):19495‐19500.
- Sun, C., P. Madsen, M. S. Lund, Y. Zhang, U. S. Nielsen, and G. Su. 2010. Improvement in genetic evaluation of female fertility in dairy cattle using multiple‐trait models including milk production traits. Journal of animal science 88:871‐878.
- Tan, W., D. F. Carlson, C. A. Lancto, J. R. Garbe, D. A. Webster, P. B. Hackett, and S. C. Fahrenkrug. 2013. Efficient nonmeiotic allele introgression in livestock using custom endonucleases. Proc. Natl. Acad. Sci. USA 110:16526‐16531.
- Thomsen, B., P. Horn, F. Panitz, E. Bendixen, A. H. Petersen, L. E. Holm, V. H. Nielsen, J. S. Agerholm, J. Arnbjerg, and C. Bendixen. 2006. A missense mutation in the bovine SLC35A3 gene, encoding a UDP‐Nacetylglucosamine transporter, causes complex vertebral malformation. Genome Res. 16:97‐105.
- VanRaden, P. M. and E. J. Klaaskate. 1993. Genetic evaluation of length of productive life including predicted longevity of live cows. J. Dairy Sci. 76:2758‐2764.
- VanRaden, P. M. and R. H. Miller. 2006. Effects of nonadditive genetic interactions, inbreeding, and recessive defects on embryo and fetal loss by seventy days. J. Dairy Sci. 89:2716‐2721.
- VanRaden, P. M., K. M. Olson, D. J. Null, and J. L. Hutchison. 2011. Harmful recessive effects on fertility detected by absence of homozygous haplotypes. J. Dairy Sci. 94:6153‐6161.
- VanRaden, P. M., A. H. Sanders, M. E. Tooker, R. H. Miller, H. D. Norman, M. T. Kuhn, and G. R. Wiggans. 2004. Development of a national genetic evaluation for cow fertility. J. Dairy Sci. 87:2285‐2292.
- Veerkamp, R. F. and B. Beerda. 2007. Genetics and genomics to improve fertility in high producing dairy cows. Theriogenology 68 (Suppl. 1):S266‐S273.
- Wang, X., V. Schutzkus, W. Huang, G. J. Rosa, and H. Khatib. 2009. Analysis of segregation distortion and association of the bovine FGF2 with fertilization rate and early embryonic survival. Anim. Genet. 40:722‐728.
- Washburn, S. P., W. J. Silvia, C. H. Brown, B. T. McDaniel, and A. J. McAllister. 2002. Trends in reproductive performance in Southeastern Holstein and Jersey DHI herds. J. Dairy Sci. 85:244‐251.
- Waters, S. M., M. S. McCabe, D. J. Howard, L. Giblin, D. A. Magee, D. E. MacHugh, and D. P. Berry. 2011. Associations between newly discovered polymorphisms in the Bos taurus growth hormone receptor gene and performance traits in HolsteinFriesian dairy cattle. Anim. Genet. 42:3949.
- Wiggans, G. R., P. M. Vanraden, and T. A. Cooper. 2011. The genomic evaluation system in the United States: past, present, future. J. Dairy Sci. 94:3202‐3211.
- Wilkening, S., B. Chen, J. L. Bermejo, and F. Canzian. 2009. Is there still a need for candidate gene approaches in the era of genome‐wide association studies? Genomics 93:415‐419.
- Wiltbank, M. C., A. H. Souza, P. D. Carvalho, A. P. Cunha, J. O. Giordano, P. M. Fricke, G. M. Baez, and M. G. Diskin. 2014. Physiological and practical effects of progesterone on reproduction in dairy cattle. Animal 8(Suppl. 1):70‐81.
- Yang, W. C., S. J. Li, K. Q. Tang, G. H. Hua, C. Y. Zhang, J. N. Yu, L. Han, and L. G. Yang. 2010.
- Polymorphisms in the 5′ upstream region of the FSH receptor gene, and their association with superovulation traits in Chinese Holstein cows. Anim. Reprod. Sci. 119:172‐177.
- Zanella, R., M. L. Settles, S. D. McKay, R. Schnabel, J. Taylor, R. H. Whitlock, Y. Schukken, J. S. Van Kessel, J. M. Smith, and H. L. Neibergs. 2011. Identification of loci associated with tolerance to Johne’s disease in Holstein cattle. Anim. Genet. 42:28‐38.
- Zhang, B., F. Penagaricano, A. Driver, H. Chen, and H. Khatib. 2011. Differential expression of heat shock protein genes and their splice variants in bovine preimplantation embryos. J. Dairy Sci. 94:4174‐4182.
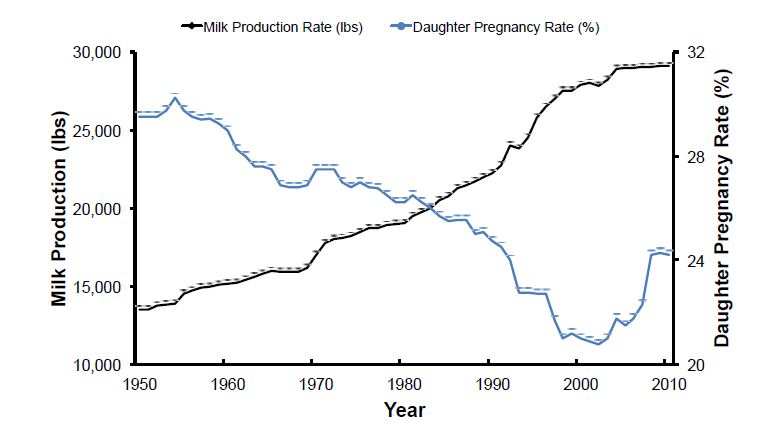
Figure 1. Association of milk production in dairy cattle with daughter pregnancy rate. Selection for milk yield in U.S. dairy cattle has been very successful to increase milk production. In contrast, cow fertility declined from 1950 to 2003 in spite of low heritabilities for reproductive traits. Fortunately, historical trends in fertility have started to recover because of the incorporation of genetic merit for productive life (PL) in 1994 and DPR in 2003 as well as the change in breeding strategies from natural service to artificial insemination and ovulation synchronization programs that decrease days open.
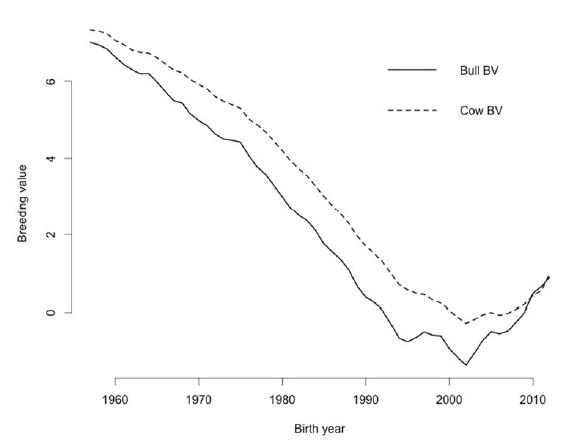
Figure 2. Historical changes in estimated breeding value (BV) for daughter pregnancy rate (DPR) in U.S. Holsteins from 1957 to 2012. Data were obtained from the USDA Animal Genomics Improvement Laboratory